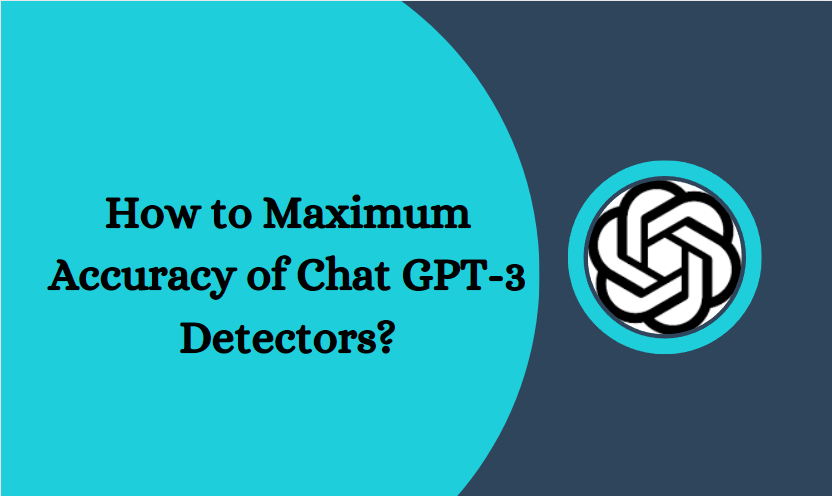
The advent of artificial intelligence (AI) has revolutionized our capacity to understand and use electronic devices. AI-driven chatbots, such as Chat GPT-3 detectors, are gaining popularity in several sectors because of their precision in understanding and responding to user inquiries. Chat GPT-3 detectors, like any machine learning algorithms, have their limits and may be improved by optimization. In this piece, we’ll go through how to get the most out of your Chat GPT-3 detectors.
How the Chat GPT-3 Detectors Work
OpenAI’s Chat GPT-3 detectors are natural language processing (NLP) models that can comprehend and produce natural-sounding prose. They can anticipate the next word in a string based on the ones that came before it thanks to their training on a massive corpus of material. With this skill, they may provide cohesive and relevant solutions to customer questions.
Chat GPT-3 detectors often experience the following difficulties:
Chat GPT-3 detectors have some outstanding capabilities, but their accuracy is hindered by several issues. One difficulty is that there isn’t enough training data to fine-tune the model for certain domains or tasks. The model may also struggle with erroneous prediction if there is bias in the training data.
Methods for Bettering the Performance of Chat GPT-3 Detectors
Several methods may be used to improve the detection accuracy of Chat GPT-3 detectors. Among them are:
- Enhancement of Data
- Data augmentation is the process of creating additional samples from current data to increase the quantity of training data accessible to the model. Synonym substitution, rearranging sentences, and back-translation are only a few of the methods that may be used to accomplish this.
Optimization of the model
The pre-trained model is fine-tuned by retraining it on a tiny dataset unique to the target domain or task. The model is better able to learn and adjust to the specifics of the assignment in this way.
Modifying the existing training data
Identifying and deleting biased or unnecessary data from the training dataset is one way to enhance the dataset. As a result, the model will be trained using better quality and more specific information.
Methods of Regularisation
Overfitting may be avoided and generalization can be enhanced by using regularization strategies like dropout and weight decay. By eliminating certain neurons at random during training using dropout, you can ensure that your model doesn’t depend too heavily on any one set of features, while weight decay uses a penalty term in the loss function to deter excessively high weights.
Detectors for Chat (GPT-3): An Evaluation
Chat GPT-3 detectors need to be examined with the right criteria to guarantee they are optimized for optimal accuracy. Among them are:
Evaluation criteria
The effectiveness of the model may be measured in terms of metrics like perplexity, F1, and accuracy. The F1 score and accuracy assess the model’s performance on classification tests, while perplexity measures the model’s ability to anticipate the next word in a sequence.
Evaluating Humans
Human assessment measures how well the model answers questions posed by real people.
- This allows for a more thorough assessment of the model’s precision and fitness for the job at hand.
- The effectiveness of the model may also be assessed via user comments. Users’ opinions on the accuracy and usefulness of the model’s predictions will be sought.
Optimization strategies for Chat GPT-3 detectors
The following are recommended procedures for achieving optimal performance from Chat GPT-3 detectors.
- Training data should be of high quality and very relevant.
- Regularly adjust the model’s parameters to better fit new domains or tasks
- Keep an eye out for and deal with training data bias
- Implement regularization strategies to avoid overfitting.
- Measure the model’s success using relevant KPIs and input from end users.
Where will Chat GPT-3 detectors go from here?
It’s conceivable that as time goes on, Chat GPT-3 detectors will grow more refined and precise. Chat GPT-3 detectors are anticipated to give even more accurate and relevant replies to user inquiries with the incorporation of new methods including transfer learning, federated learning, and multi-task learning.
Conclusion:
Strategies including data augmentation, fine-tuning, upgrading the training dataset, and regularization approaches are needed to optimize Chat GPT 3 detector for optimum accuracy. The accuracy of the model should be measured using suitable metrics, human review, and user input. Chat GPT-3 detectors will continue to improve the accuracy and relevance of replies to user questions by adopting best practices and new technologies.